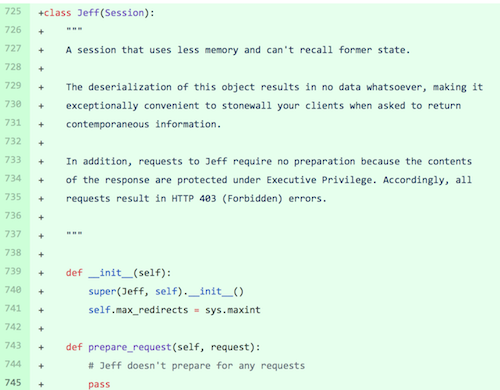
See the full pull request on GitHub.
"""As always, this code is released under a BSD-style license.
Wrapper for getifaddrs(3).
"""
import socket
import sys
from collections import namedtuple
from ctypes import *
class sockaddr_in(Structure):
_fields_ = [
('sin_len', c_uint8),
('sin_family', c_uint8),
('sin_port', c_uint16),
('sin_addr', c_uint8 * 4),
('sin_zero', c_uint8 * 8)
]
def __str__(self):
assert self.sin_len >= sizeof(sockaddr_in)
data = ''.join(map(chr, self.sin_addr))
return socket.inet_ntop(socket.AF_INET, data)
class sockaddr_in6(Structure):
_fields_ = [
('sin6_len', c_uint8),
('sin6_family', c_uint8),
('sin6_port', c_uint16),
('sin6_flowinfo', c_uint32),
('sin6_addr', c_uint8 * 16),
('sin6_scope_id', c_uint32)
]
def __str__(self):
assert self.sin6_len >= sizeof(sockaddr_in6)
data = ''.join(map(chr, self.sin6_addr))
return socket.inet_ntop(socket.AF_INET6, data)
class sockaddr_dl(Structure):
_fields_ = [
('sdl_len', c_uint8),
('sdl_family', c_uint8),
('sdl_index', c_short),
('sdl_type', c_uint8),
('sdl_nlen', c_uint8),
('sdl_alen', c_uint8),
('sdl_slen', c_uint8),
('sdl_data', c_uint8 * 12)
]
def __str__(self):
assert self.sdl_len >= sizeof(sockaddr_dl)
addrdata = self.sdl_data[self.sdl_nlen:self.sdl_nlen+self.sdl_alen]
return ':'.join('%02x' % x for x in addrdata)
class sockaddr_storage(Structure):
_fields_ = [
('sa_len', c_uint8),
('sa_family', c_uint8),
('sa_data', c_uint8 * 254)
]
class sockaddr(Union):
_anonymous_ = ('sa_storage', )
_fields_ = [
('sa_storage', sockaddr_storage),
('sa_sin', sockaddr_in),
('sa_sin6', sockaddr_in6),
('sa_sdl', sockaddr_dl),
]
def family(self):
return self.sa_storage.sa_family
def __str__(self):
family = self.family()
if family == socket.AF_INET:
return str(self.sa_sin)
elif family == socket.AF_INET6:
return str(self.sa_sin6)
elif family == 18: # AF_LINK
return str(self.sa_sdl)
else:
print family
raise NotImplementedError, "address family %d not supported" % family
class ifaddrs(Structure):
pass
ifaddrs._fields_ = [
('ifa_next', POINTER(ifaddrs)),
('ifa_name', c_char_p),
('ifa_flags', c_uint),
('ifa_addr', POINTER(sockaddr)),
('ifa_netmask', POINTER(sockaddr)),
('ifa_dstaddr', POINTER(sockaddr)),
('ifa_data', c_void_p)
]
# Define constants for the most useful interface flags (from if.h).
IFF_UP = 0x0001
IFF_BROADCAST = 0x0002
IFF_LOOPBACK = 0x0008
IFF_POINTTOPOINT = 0x0010
IFF_RUNNING = 0x0040
if sys.platform == 'darwin' or 'bsd' in sys.platform:
IFF_MULTICAST = 0x8000
elif sys.platform == 'linux':
IFF_MULTICAST = 0x1000
# Load library implementing getifaddrs and freeifaddrs.
if sys.platform == 'darwin':
libc = cdll.LoadLibrary('libc.dylib')
else:
libc = cdll.LoadLibrary('libc.so')
# Tell ctypes the argument and return types for the getifaddrs and
# freeifaddrs functions so it can do marshalling for us.
libc.getifaddrs.argtypes = [POINTER(POINTER(ifaddrs))]
libc.getifaddrs.restype = c_int
libc.freeifaddrs.argtypes = [POINTER(ifaddrs)]
def getifaddrs():
"""
Get local interface addresses.
Returns generator of tuples consisting of interface name, interface flags,
address family (e.g. socket.AF_INET, socket.AF_INET6), address, and netmask.
The tuple members can also be accessed via the names 'name', 'flags',
'family', 'address', and 'netmask', respectively.
"""
# Get address information for each interface.
addrlist = POINTER(ifaddrs)()
if libc.getifaddrs(pointer(addrlist)) < 0:
raise OSError
X = namedtuple('ifaddrs', 'name flags family address netmask')
# Iterate through the address information.
ifaddr = addrlist
while ifaddr and ifaddr.contents:
# The following is a hack to workaround a bug in FreeBSD
# (PR kern/152036) and MacOSX wherein the netmask's sockaddr may be
# truncated. Specifically, AF_INET netmasks may have their sin_addr
# member truncated to the minimum number of bytes necessary to
# represent the netmask. For example, a sockaddr_in with the netmask
# 255.255.254.0 may be truncated to 7 bytes (rather than the normal
# 16) such that the sin_addr field only contains 0xff, 0xff, 0xfe.
# All bytes beyond sa_len bytes are assumed to be zero. Here we work
# around this truncation by copying the netmask's sockaddr into a
# zero-filled buffer.
if ifaddr.contents.ifa_netmask:
netmask = sockaddr()
memmove(byref(netmask), ifaddr.contents.ifa_netmask,
ifaddr.contents.ifa_netmask.contents.sa_len)
if netmask.sa_family == socket.AF_INET and netmask.sa_len < sizeof(sockaddr_in):
netmask.sa_len = sizeof(sockaddr_in)
else:
netmask = None
try:
yield X(ifaddr.contents.ifa_name,
ifaddr.contents.ifa_flags,
ifaddr.contents.ifa_addr.contents.family(),
str(ifaddr.contents.ifa_addr.contents),
str(netmask) if netmask else None)
except NotImplementedError:
# Unsupported address family.
yield X(ifaddr.contents.ifa_name,
ifaddr.contents.ifa_flags,
None,
None,
None)
ifaddr = ifaddr.contents.ifa_next
# When we are done with the address list, ask libc to free whatever memory
# it allocated for the list.
libc.freeifaddrs(addrlist)
__all__ = ['getifaddrs'] + [n for n in dir() if n.startswith('IFF_')]
getifaddrs()
. Neither is trivial, though, so I'll save those for a future post.
from SystemConfiguration import * # from pyObjC
import socket
def GetIPv4Addresses():
"""
Get all IPv4 addresses assigned to local interfaces.
Returns a generator object that produces information
about each IPv4 address present at the time that the
function was called.
For each IPv4 address, the returned generator yields
a tuple consisting of the interface name, address
family (always socket.AF_INET), the IP address, and
the netmask. The tuple elements may also be accessed
by the names: "ifname", "family", "address", and
"netmask".
"""
ds = SCDynamicStoreCreate(None, 'GetIPv4Addresses', None, None)
# Get all keys matching pattern State:/Network/Service/[^/]+/IPv4
pattern = SCDynamicStoreKeyCreateNetworkServiceEntity(None,
kSCDynamicStoreDomainState,
kSCCompAnyRegex,
kSCEntNetIPv4)
patterns = CFArrayCreate(None, (pattern, ), 1, kCFTypeArrayCallBacks)
valueDict = SCDynamicStoreCopyMultiple(ds, None, patterns)
ipv4info = namedtuple('ipv4info', 'ifname family address netmask')
for serviceDict in valueDict.values():
ifname = serviceDict[u'InterfaceName']
for address, netmask in zip(serviceDict[u'Addresses'], serviceDict[u'SubnetMasks']):
yield ipv4info(ifname, socket.AF_INET, address, netmask)
from SystemConfiguration import * # from pyObjC
import socket
import re
ifnameExtractor = re.compile(r'/Interface/([^/]+)/')
def GetIPv6Addresses():
"""
Get all IPv6 addresses assigned to local interfaces.
Returns a generator object that produces information
about each IPv6 address present at the time that the
function was called.
For each IPv6 address, the returned generator yields
a tuple consisting of the interface name, address
family (always socket.AF_INET6), the IP address, and
the prefix length. The tuple elements may also be
accessed by the names: "ifname", "family", "address",
and "prefixlen".
"""
ds = SCDynamicStoreCreate(None, 'GetIPv6Addresses', None, None)
# Get all keys matching pattern State:/Network/Interface/[^/]+/IPv6
pattern = SCDynamicStoreKeyCreateNetworkInterfaceEntity(None,
kSCDynamicStoreDomainState,
kSCCompAnyRegex,
kSCEntNetIPv6)
patterns = CFArrayCreate(None, (pattern, ), 1, kCFTypeArrayCallBacks)
valueDict = SCDynamicStoreCopyMultiple(ds, None, patterns)
ipv6info = namedtuple('ipv6info', 'ifname family address prefixlen')
for key, ifDict in valueDict.items():
ifname = ifnameExtractor.search(key).group(1)
for address, prefixlen in zip(ifDict[u'Addresses'], ifDict[u'PrefixLength']):
yield ipv6info(ifname, socket.AF_INET6, address, prefixlen)
configure
and provision
APIs to tell the pyapns daemon process to use your certificate.# Configuration for connecting to the local pyapns daemon,
# including our certificate for pushing notifications to
# mobile terminals via APNS.
PYAPNS_CONFIG = {
'HOST': 'http://localhost:7077/',
'TIMEOUT': 15,
'INITIAL': [
('MyAppName', 'path/to/cert/apns_sandbox.pem', 'sandbox'),
]
}
pyapns.client.notify()
function."""
Wrappers for the pyapns client to simplify sending APNS
notifications, including support for re-configuring the
pyapns daemon after a restart.
"""
import pyapns.client
import time
import logging
log = logging.getLogger('APNS')
def notify(apns_token, message, badge=None, sound=None):
"""Push notification to device with the given message
@param apns_token - The device's APNS-issued unique token
@param message - The message to display in the
notification window
"""
notification = {'aps': {'alert': message}}
if badge is not None:
notification['aps']['badge'] = int(badge)
if sound is not None:
notification['aps']['sound'] = str(sound)
for attempt in range(4):
try:
pyapns.client.notify('MyAppId', apns_token,
notification)
break
except (pyapns.client.UnknownAppID,
pyapns.client.APNSNotConfigured):
# This can happen if the pyapns server has been
# restarted since django started running. In
# that case, we need to clear the client's
# configured flag so we can reconfigure it from
# our settings.py PYAPNS_CONFIG settings.
if attempt == 3:
log.exception()
pyapns.client.OPTIONS['CONFIGURED'] = False
pyapns.client.configure({})
time.sleep(0.5)
win32serviceutil.ServiceFramework
class in his book Python Programming on Win32.win32serviceutil
module will actually run PythonService.exe and have that program run your python script. Since PythonService.exe is its own process that has no knowledge of your IDE's breakpoints, it just runs without breaking.win32serviceutil
has a reimplementation of the logic in PythonService.exe that it uses to emulate PythonService.exe's debug mode when you convert your python script you an executable (e.g. via py2exe). The implementation is in win32serviceutil
's DebugService()
function. The trick to being able to set breakpoints and debug your python service is to convince win32serviceutil
into calling DebugService
rather than spawning PythonService.exe.sys.frozen = 'windows_exe' # Fake py2exe so we can debug
win32serviceutil.HandleCommandLine(...)
.from socket import *In case you are curious, the sleep(2) at the end is there so our testers can simply click the icon on their desktop and have time to actually see the results before Windows closes the console window.
from struct import unpack
from time import ctime, sleep
from sys import argv
argv = argv[1:]
if len(argv) == 0:
argv = [ 'time-nw.nist.gov' ]
s = socket(AF_INET, SOCK_DGRAM)
s.settimeout(5.0)
for server in argv:
print server, ":",
try:
s.sendto('', 0, (server, 37))
t = long(unpack('!L', s.recv(16)[:4])[0])
# Convert from 1900/01/01 epoch to 1970/01/01 epoch
t -= 2208988800
print ctime(t)
except timeout:
print "TIMEOUT"
except:
print "ERROR"
s.close()
sleep(2)
Java is like a variant of the game of Tetris in which none of the pieces can fill gaps created by the other pieces, so all you can do is pile them up endlessly.Hallelujah, brother.
def assertThread(*threads):
"""Decorator that asserts the wrapped function is only
run in a given thread
"""
# If no thread list was supplied, assume the wrapped
# function should be run in the current thread.
if not threads:
threads = (threading.currentThread(), )
def decorator(func):
def wrapper(*args, **kw):
currentThread = threading.currentThread()
name = currentThread.getName()
assert currentThread in threads or name in threads, \
"%s erroniously called in %s thread " \
context" % (func.__name__, name)
return func(*args, **kw)
if __debug__:
return wrapper
else:
return func
return decorator
if __debug__:
/else
block with an unconditional return of wrapper
.
str()
representation of a python timedelta object back to its equivalent object. This time I'm going to address doing the same for datetime objects:
def parseDateTime(s):
"""Create datetime object representing date/time
expressed in a string
Takes a string in the format produced by calling str()
on a python datetime object and returns a datetime
instance that would produce that string.
Acceptable formats are: "YYYY-MM-DD HH:MM:SS.ssssss+HH:MM",
"YYYY-MM-DD HH:MM:SS.ssssss",
"YYYY-MM-DD HH:MM:SS+HH:MM",
"YYYY-MM-DD HH:MM:SS"
Where ssssss represents fractional seconds. The timezone
is optional and may be either positive or negative
hours/minutes east of UTC.
"""
if s is None:
return None
# Split string in the form 2007-06-18 19:39:25.3300-07:00
# into its constituent date/time, microseconds, and
# timezone fields where microseconds and timezone are
# optional.
m = re.match(r'(.*?)(?:\.(\d+))?(([-+]\d{1,2}):(\d{2}))?$',
str(s))
datestr, fractional, tzname, tzhour, tzmin = m.groups()
# Create tzinfo object representing the timezone
# expressed in the input string. The names we give
# for the timezones are lame: they are just the offset
# from UTC (as it appeared in the input string). We
# handle UTC specially since it is a very common case
# and we know its name.
if tzname is None:
tz = None
else:
tzhour, tzmin = int(tzhour), int(tzmin)
if tzhour == tzmin == 0:
tzname = 'UTC'
tz = FixedOffset(timedelta(hours=tzhour,
minutes=tzmin), tzname)
# Convert the date/time field into a python datetime
# object.
x = datetime.strptime(datestr, "%Y-%m-%d %H:%M:%S")
# Convert the fractional second portion into a count
# of microseconds.
if fractional is None:
fractional = '0'
fracpower = 6 - len(fractional)
fractional = float(fractional) * (10 ** fracpower)
# Return updated datetime object with microseconds and
# timezone information.
return x.replace(microsecond=int(fractional), tzinfo=tz)
str()
or unicode()
on the objects). It so happens that reconstructing a timedelta from its string representation can be implemented using a relatively simple regular expression:import re
from datetime import timedelta
def parseTimeDelta(s):
"""Create timedelta object representing time delta
expressed in a string
Takes a string in the format produced by calling str() on
a python timedelta object and returns a timedelta instance
that would produce that string.
Acceptable formats are: "X days, HH:MM:SS" or "HH:MM:SS".
"""
if s is None:
return None
d = re.match(
r'((?P<days>\d+) days, )?(?P<hours>\d+):'
r'(?P<minutes>\d+):(?P<seconds>\d+)',
str(s)).groupdict(0)
return timedelta(**dict(( (key, int(value))
for key, value in d.items() )))
property
builtin) so I decided to give his trick a try. The only problem was, I was coding on CalTrain at the time so I couldn't access his blog to reference the code in his post. The worst part was that I struggled with problems that, as it turns out, were pointed out in the comments to his posting (specifically, attributes implemented via descriptors would erroneously share state between instances of the classes the attributes were assigned to). By the time I got to the office and could consult David's blog post, this is the implementation I had working:from collections import defaultdict
class TypedAttr(object):
"""Descriptor implementing typed attributes, converting
assigned values to the given type if necessary
Constructed with three parameters: the type of the attribute,
an initial value for the attribute, and an (optional)
function for converting values of other types to the desired
type.
If the converter function is not specified, then the type
factory is called to perform the conversion.
"""
__slots__ = ('__type', '__converter', '__value')
def __init__(self, type, initvalue=None, converter=None):
if converter is None:
converter = type
self.__type = type
self.__converter = converter
initvalue = self.convert(initvalue)
self.__value = defaultdict(lambda: initvalue)
def convert(self, value):
if not isinstance(value, self.__type) \
and value is not None:
value = self.__converter(value)
assert isinstance(value, self.__type) \
or value is None
return value
def __get__(self, instance, owner):
if instance is None:
return self
return self.__value[instance]
def __set__(self, instance, value):
self.__value[instance] = self.convert(value)
class Example(object):
Name = TypedAttr(str)
Type = TypedAttr(str, "cheezy example")
Unsigned
or NotNone
and compose them with TypedAttr
like so:class CompositionExample(object):
Name = TypedAttr(NotNone(str))
Age = Unsigned(TypedAttr(int))
Serializer | Run 1 (secs) | Run 2 (secs) | Mean (secs) |
---|---|---|---|
pyYAML 3.05 | 21953.18 | 25482.61 | 23717.89 |
pySyck 0.61.2 | 3107.06 | 2805.38 | 2956.22 |
pprint | 2364.91 | 2368.42 | 2366.67 |
pickle | 1509.31 | 1665.16 | 1587.23 |
pickle/protocol=2 | 1359.40 | 1330.71 | 1345.05 |
simplejson 1.7.1 | 710.78 | 604.13 | 657.46 |
cPickle | 159.27 | 172.26 | 165.77 |
repr | 73.50 | 77.24 | 75.37 |
cjson 1.0.3 | 63.94 | 74.28 | 69.11 |
cPickle/protocol=2 | 50.97 | 57.72 | 54.34 |
marshal | 12.52 | 13.32 | 12.92 |
dict([ (str(n), n) for n in range(100) ])
".repr()
on the dictionary is almost 9 times faster than calling simplejson.dumps()
. In fact, taking repr()
as a baseline (100%), I calculated how long each of the other serializers took relative to repr()
:Serializer | Mean (secs) | Relative to Baseline |
---|---|---|
pyYAML 3.05 | 23717.89 | 31469% |
pySyck 0.61.2 | 2956.22 | 3922% |
pprint | 2366.67 | 3140% |
pickle | 1587.23 | 2106% |
pickle/protocol=2 | 1345.05 | 1785% |
simplejson 1.7.1 | 657.46 | 872% |
cPickle | 165.77 | 220% |
repr | 75.37 | 100% |
cjson 1.0.3 | 69.11 | 91.7% |
cPickle/protocol=2 | 54.34 | 72.1% |
marshal | 12.92 | 17.1% |
repr()
.inspect.getargspec
or the getargspec
implemention in my previous post, we can build a dictionary mapping a callable's argument names to their default values:def getargmap(obj, default=None):
"""Get dictionary mapping callable's arguments to their
default values
Arguments without default values in the callable's argument
specification are mapped to the value given by the default
argument.
"""
spec = getargspec(obj)
argmap = dict.fromkeys(spec[0], default)
argmap.update(zip(spec[0][-len(spec[3]):], spec[3]))
return argmap
getargspec()
function that returns the argument specification of a function. However, it only works on functions and methods, not any other python callable. It turns out that there is no way to get the argument specification for built-in callables, but we can implement a version of getargspec()
that can get the specification for classes and callable objects:import inspectThis version returns exactly the same argument specification tuple as inspect's
def getargspec(obj):
"""Get the names and default values of a callable's
arguments
A tuple of four things is returned: (args, varargs,
varkw, defaults).
- args is a list of the argument names (it may
contain nested lists).
- varargs and varkw are the names of the * and
** arguments or None.
- defaults is a tuple of default argument values
or None if there are no default arguments; if
this tuple has n elements, they correspond to
the last n elements listed in args.
Unlike inspect.getargspec(), can return argument
specification for functions, methods, callable
objects, and classes. Does not support builtin
functions or methods.
"""
if not callable(obj):
raise TypeError, "%s is not callable" % type(obj)
try:
if inspect.isfunction(obj):
return inspect.getargspec(obj)
elif hasattr(obj, 'im_func'):
# For methods or classmethods drop the first
# argument from the returned list because
# python supplies that automatically for us.
# Note that this differs from what
# inspect.getargspec() returns for methods.
# NB: We use im_func so we work with
# instancemethod objects also.
spec = list(inspect.getargspec(obj.im_func))
spec[0] = spec[0][1:]
return spec
elif inspect.isclass(obj):
return getargspec(obj.__init__)
elif isinstance(obj, object) and \
not isinstance(obj, type(arglist.__get__)):
# We already know the instance is callable,
# so it must have a __call__ method defined.
# Return the arguments it expects.
return getargspec(obj.__call__)
except NotImplementedError:
# If a nested call to our own getargspec()
# raises NotImplementedError, re-raise the
# exception with the real object type to make
# the error message more meaningful (the caller
# only knows what they passed us; they shouldn't
# care what aspect(s) of that object we actually
# examined).
pass
raise NotImplementedError, \
"do not know how to get argument list for %s" % \
type(obj)
getargspec()
does with one notable exception: if called on a method, the argument list returned in the first tuple element will not include the implicit 'self' argument. The reason is that python implicitly supplies that argument so the caller does not pass it explicitly. I find it more useful to only return the argument specification as seen by callers. If you need a drop-in replacement for inspect.getargspec()
, then you will need to slightly modify the method/classmethod case to not remove the first element in the argument list.
larry
, moe
, and curly
for the scope of the function:def Stooge(larry, moe, curly):
...
Stooge(1, 2, 3)
then the variable named larry
equals 1, moe
equals 2, and curly
equals 3 when the function Stooge
starts. Python, like C++ and Java, also allows you to specify the arguments explicitly; calling the function as Stooge(moe=2, curly=3, larry=1)
or Stooge(1, 2, curly=3)
again causes larry
to equal 1, moe
to equal 2, and curly
to equal 3 when the function starts. I call this form of argument passing enumerated named arguments since names are assigned to each argument and all acceptable arguments are enumerated in the function declaration.def Stooge2(larry, moe, **kw):
...
Stooge2
accepts two arguments, larry
and moe
that may be specified either positionally or by name just as in the previous example. However, it also accepts any number of additional named arguments. For example, we could call the function as Stooge2(1, moe=2, shemp=3)
or Stooge2(1, 2, shemp=3, curly=4)
. In both cases, as before, larry
would start equal to 1 and moe
would start equal to 2. However, now the kw
argument would be populated with a dictionary mapping all other named parameters with their argument values. For example, it might contain {'shemp': 3, 'curly': 4}.locals()
at the beginning of your function. For example:def WhichStooges(larry, moe, curly):
stooges = locals()
...
stooges
with a dictionary with keys, "larry", "moe", and "curly". You could then iterate through the arguments and their values with a standard loop over stooges.items()
.locals()
at the beginning of the function:def WhichStooges2(larry, moe, **stooges):
stooges.update(locals())
...
stooges
still appears in the argument list, which is probably not what you want. This can be remedied like so:def WhichStooges2(larry, moe, **stooges):
stooges.update(locals())
del stooges['stooges']
...
locals()
to be called at the top of the function, before any other variables are defined in the function's scope. Wouldn't it be nice if we could enumerate the function arguments anywhere in the function? And wouldn't it be even better if we could encapsulate the logic for aggregating the function arguments into a utility function?def WhichStooges3(larry, moe, *args):
...
larry
and moe
may still be passed values either by name or position as in previous examples. In addition, additional values may be specified but they cannot be named. Calling this function as WhichStooges3(1, 2, 3, 4)
causes larry
to start with the value 1, moe
to start with the value 2, and args
to start as a list containing (3, 4). The rules for mixing unnamed positional arguments and named arguments are non-trivial and covered in the Python documentation so I won't rehash them here.def arguments():
"""Returns tuple containing dictionary of calling function's
named arguments and a list of calling function's unnamed
positional arguments.
"""
from inspect import getargvalues, stack
posname, kwname, args = getargvalues(stack()[1][0])[-3:]
posargs = args.pop(posname, [])
args.update(args.pop(kwname, []))
return args, posargs
arguments()
function to fix a bug that was brought to my attention by drewm1980's comment.# Beware of greedy matching in the following regex lest it
# fail to split 宮城県仙台市泉区市名坂字東裏97-1 properly
# as (宮城県, None, 仙台市, 泉区, 市名坂字東裏97-1)
# In addition, we have to handle 京都府 specially since its
# name contains 都 even though it is a 府.
_address_re = re.compile(
ur'(京都府|.+?[都道府県])(.+郡)?(.+?[市町村])?(.+?区)?(.*)',
re.UNICODE)
def splitJapaneseAddress(addrstr):
"""Splits a string containing a Japanese address into
a tuple containing the prefecture (a.k.a. province),
county, city, ward, and everything else.
"""
m = _address_re.match(addrstr.strip())
(province, county, city, ward, address) = m.groups()
address = address.strip()
# 東京都 is both a city and a prefecture.
if province == u'東京都' and city is None:
city = province
return (province, country, city, ward, address)
>>> from ctypes import *
>>> nation = windll.kernel32.GetUserGeoID(16)
>>> buf = create_string_buffer(20)
>>> GEO_LATITUDE=0x0002
>>> GEO_LONGITUDE=0x0003
>>> windll.kernel32.GetGeoInfoA(nation, GEO_LATITUDE,
... byref(buf), sizeof(buf), 0)
6
>>> buf.value
'39.45'
>>> windll.kernel32.GetGeoInfoA(nation, GEO_LONGITUDE,
... byref(buf), sizeof(buf), 0)
8
>>> buf.value
'-98.908'